In a fit of pique, Clem Delangue began live-tweeting. He was packed inside a lecture hall at the University College in Dublin, where Delangue was continuing a hopscotch of study abroad posts, from his full-time university in Paris, to Madrid, to Bangalore, and now in the fall of 2011 to Ireland. “Peter O’Neill, Country General Manager for IBM Ireland on stage @ucddublin for a guest lecture,” he typed, and hit tweet. It was 9:01 AM on a Tuesday. Everyone was facing the same direction, hunched over notebooks and laptops. Delangue, frustrated to be staring silently at the backs of heads, was doing his best to tweet his way to some kind of connection.
Lectures like this, with hundreds of students taking identical notes, struck Delangue as a hived-off relic of pre-internet thinking. “Why would we be doing that,” he thought, “without sharing with each other. Without sharing externally?” They weren’t just missing out on collaboration within the room; they were also missing an opportunity to share insights with a community of students around the world who could only dream of such an education.
Back in his dorm that night, Delangue began work on a solution to what he considered to be a fundamental, unjust bottleneck in how learning is distributed worldwide. The following summer, a 23-year-old Delangue was on a stage in Paris, delivering a TEDx Talk about his platform, UniShared—his effort to connect classmates and offer the benefits of a college education to everyone on the planet. “The classroom is a bubble,” he told that audience in Paris. “Whereas it should be a place for openness to the world, to others. I’ve never felt so isolated than in a classroom.”
Delangue knows a thing or two about isolation. Years before he created his open-source education software, Delangue’s world didn’t extend much beyond the borders of La Bassée, his home town on the Northern tip of France, that Delangue remembers as “remote, with barely anything to do.” The third child of four to a nurse and a lawnmower shop owner, Delangue always felt that he played the role of connector and peacekeeper in his family. Travel was a challenge, logistically and financially, with a family of six, so Delangue’s opportunities for exploration were limited. That is, until the internet arrived. “When I got my first computer,” he remembers, “it was kind of like a window into the rest of the world that was, you know, mind-blowing.”
Not only did the internet expand Delangue’s horizons, it also created opportunities for the budding entrepreneur that the ‘real’ world simply couldn’t. Online, he and his older brother set up shop on eBay and sold hundreds of imported ATVs and motorbikes. Yet when 16-year-old Delangue stood in his father’s store trying to sell tractors to grown men, he knew they didn’t take him seriously. “The internet was creating new rules for me,” he says.
Clem’s platform, UniShared, didn’t knock the world off its axis (it remains available as an open source tool). But it did help ensure that when he graduated college in 2012, he had a job offer at Google waiting for him. Ultimately he declined, already feeling more at home in smaller, nimbler environments. He instead went to work for a Paris-based computer vision startup called Moodstocks. The company was building an app that allowed a user to scan an object and immediately see relevant reviews and e-commerce portals. See a book, point your phone at it, and buy it in an instant. This was Delangue’s first flirtation with the then-niche realm of machine learning—and it felt like a revelation.
A chance meeting at a conference in Paris with the famed Apple marketer Guy Kawasaki cemented for Delangue the power of this tech. Delangue approached Kawasaki as he was signing books (young Delangue is center-frame in this video of the event) and introduced himself and his app. Kawasaki was skeptical of the technology—specifically its ability to identify random objects in the wild, a revolutionary claim at a time when ML still relied on engineers to specify a relatively short, static list of permitted definitions and rules in order to get accurate results. “He said something like, there’s no way you can create rules to recognize the total of objects in the world,” says Delangue.
So Delangue offered Kawasaki a demo, pointing his smartphone at Kawasaki’s bottle of water. To Kawasaki’s surprise, the app correctly identified the brand and pulled up social reviews, including an effusive comment from one of Delangue’s colleagues at Moodstocks. The true electricity of that moment for Delangue wasn’t just that his app worked. Rather, it was the potential he saw for machine learning to create connections between people in new ways. “The two people who have never met—one French geeky engineer and this American Silicon Valley guy—they discovered, thanks to machine learning, they actually like the same water brand.” Delangue says. To be sure, ML had incredible potential—but even more so when developed with a community of users in mind.
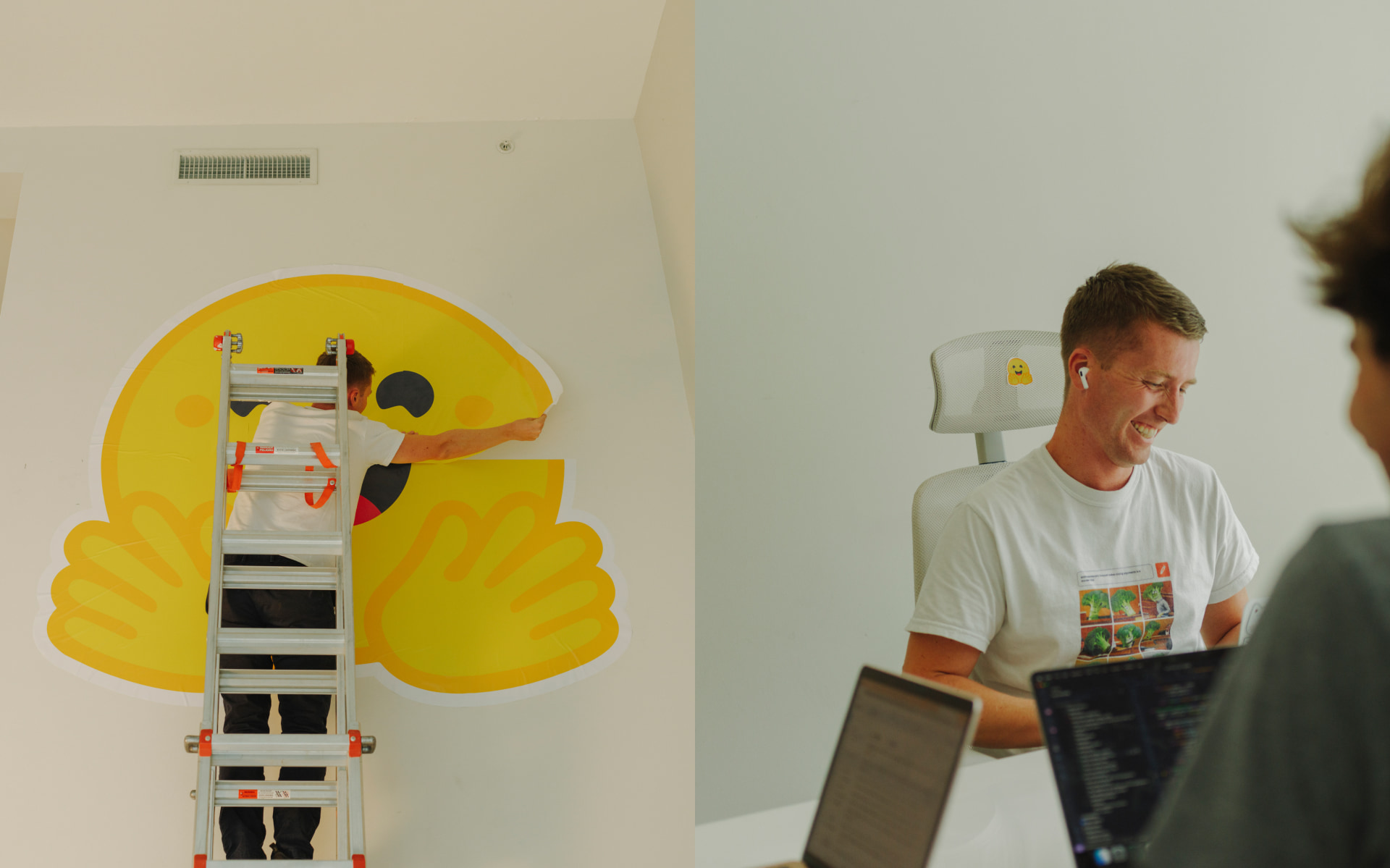
Clem shows up to our video interview from his home in North Miami Beach wearing a T-shirt with a screen-printed grid of nine images depicting a man mowing the iconic grassy field from the old Windows XP wallpaper. The pictures were generated, he tells me, by Dall-E Mini, an explosively popular text-to-image AI tool that Delangue also used to explain AI to his father. “I told him, ‘OK, tell me anything, and the AI will make it.’ And he said, ‘a John Deere tractor, on the moon.’” So, father and son got to bond over heavy machinery in space, seemingly placed there by some impossible miracle, or perhaps by a superhuman effort (which, when you consider the technology underlying the image, is not so far from the truth).
It was a swing at the impossible that put Hugging Face on its current trajectory as the home for the world’s largest community of open source AI builders. After leaving Moodstocks in 2012 (four years before Google acquired it), Delangue worked in product and marketing for a handful of startups that all got acquired. By 2016, he was itching to build something for himself again. In New York City he linked up with Julien Chaumond (a computer engineer who was working in France’s economic ministry). He’d known Julien, an elite mathematician and friend with whom he’d had a mutual professional crush, for years. The two decided to take an online Stanford engineering class together, and, true to form, surrounded themselves with a study group of three dozen people. Among them was Thomas Wolf (a trained scientist turned patent lawyer), who played in a band with Julien. By the time the class finished, Julien and Clem had invited Thomas to come work with them.
Together they set out to solve one of the most difficult problems they could identify in the burgeoning field of machine learning: The trio aimed to leverage a technology called natural language processing (NLP) to build an open-domain, conversational AI chatbot. One that could talk with you about anything. Siri had been around since 2011; Alexa, since 2014. “They were extremely boring,” Delangue says. “They would only do productivity stuff. We got excited by the idea of building a fun conversational AI.”
“We got excited by the idea of building a fun conversational AI.”
Clem Delangue
In 2023 that sounds like an attainable—perhaps even obvious—goal. But in 2016, the field of deep learning for NLP (that is, NLP that relied on deep neural networks for learning rather than the statistical, rule-based NLP of the past) was so nascent that their objective was borderline sci-fi. To create the sort of digital companion they were after, the chatbot would have to incorporate multiple learning models, to perform information extraction, to understand sentiment, and to generate answers. And for those models to function, they’d need to train them on unruly quantities of data. Managing that data—figuring out how to gather enough of it, label it, host it—was their first major challenge. Long before Hugging Face could become a nerve center for AI development, it had to address these core difficulties of the ML space.
From the jump, the Hugging Face team displayed a certain spirit of generosity and democracy that positioned them for success. It began with their employees. Delangue and his co-founders empowered them to take ownership of their product, creating and shipping features for their chatbot. Eventually that bot lived on social, mobile and the web, and its users exchanged more than a billion messages. Over time, it embraced an open-source first approach.
No moment better encapsulated this ethos than when Hugging Face released its own custom version of an ML model called BERT (short for Bidirectional Encoder Representations from Transformers). At the time, advances in machine learning tended to arrive via dense, theoretical academic articles. Similarly, when Google first released BERT in October 2018, it was too complex for most users—compounded by the fact that BERT was available only on Google’s TensorFlow platform. The Hugging Face crew decided that BERT needed to be more widely accessible. Within a week, they’d created a working version that used the machine learning framework PyTorch. Then they made their conversion open-source, giving it away on Github to anyone who wanted to use it, or modify it further.
“That was a watershed moment,” says Pat Grady, a Sequoia partner who works with Hugging Face. “People were like, ‘Oh my God, I can use a cutting edge language model.’ It just hadn’t been possible before. That made Hugging Face heroes within what was then the very small community of natural language processing people.” When Grady later asked Delangue what inspired them to release BERT to the world, he was struck by the straightforwardness of his answer. “It just seemed to be something a lot of people would like to use,” Delangue replied.
The response to BERT within the ML community reaffirmed what Delangue had felt years earlier in his lecture hall in Dublin—that sharing knowledge benefits everybody. That’s when the mission at Hugging Face changed. Delangue and his co-founders decided to start sharing everything that they had learned about ML in the process of building their chatbot. Gradually, they started to become a go-to resource for engineers, researchers and hobbyists in the machine learning space.
In the process of building their BERT adaptation, Delangue and his team became experts on a new model called a transformer. All AI models are trained on massive amounts of data, and transformers were capable of generating excellent results from unlabeled data (e.g., no longer would a computer vision system need a human to label an image as a cat, as it could learn to identify cats on its own). This form of unsupervised learning saves data curators massive amounts of time, and makes much more data available for training ML systems.
The Hugging Face team saw transformers’ potential and established Hugging Face as the go-to open-source hub to develop them. And the community took note—coders and researchers started flocking to Hugging Face, cross-pollinating an abundance of ML projects and tools with fresh insights and expertise. Today, anyone who wants to build with transformers can access some 200,000 different public models in Hugging Face’s hub.
Delangue realized that given the vast potential of these tools, making sense of them takes a village: people who organize around common goals, needs and values. With their move to open-source, Hugging Face was starting to become the community Delangue had hoped he would someday build.
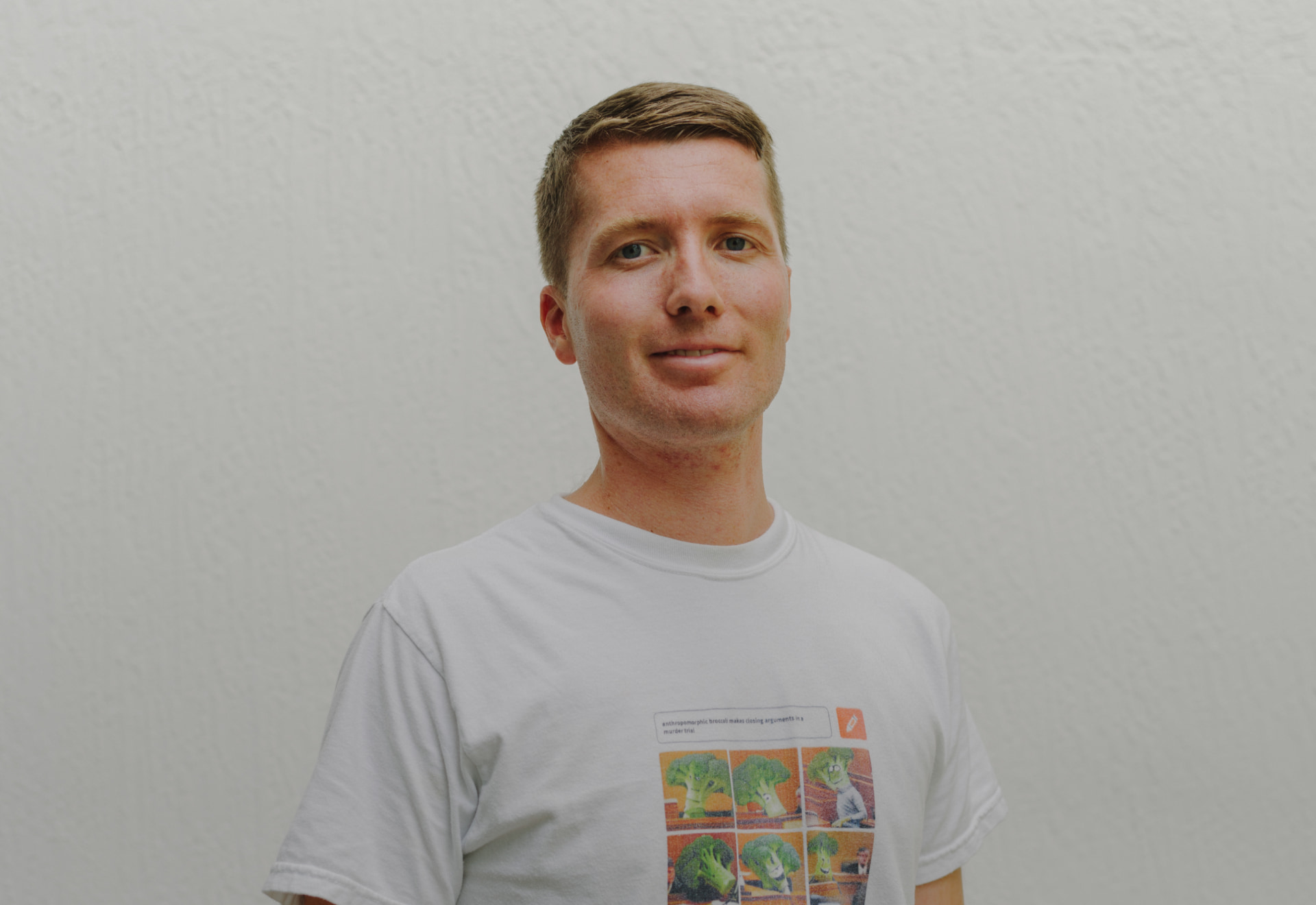
“We felt like if we started to have community managers, it would be outsourcing a responsibility that all members must keep,”
Clem Delangue
“It was pretty obvious there was something happening with the company,” says Sequoia Partner Sonya Huang. “Very few people are actually extraordinary at creating communities. Delangue understands the user—he is the user—and he has this intuition for grassroots community. He nurtures it so authentically.”
Huang was also struck by the way Delangue seemed to walk the open-source walk. She notes that instead of hiring a dedicated community manager to interface with Hugging Face’s users, Delangue did it himself, routinely tweeting bug fixes and troubleshooting features with more than 1,000 AI researchers and 10,000 companies chasing AI breakthroughs in biotech, imaging, and language processing.
To this day Hugging Face not only has no single community manager, every one of its 160 employees are expected to be active participants in this online community. “We felt like if we started to have community managers, it would be outsourcing a responsibility that all members must keep,” says Delangue. To that end, every employee has access to the company’s official Twitter and LinkedIn accounts. If the future of AI is to be crowdsourced, Delangue is ensuring that Hugging Face has positioned itself as the source of the crowd.
Today, the company is a go-to hub for machine learning. The sheer size of the Hugging Face community feeds the inertia of its strategic position. It has become the place where everyone goes for everything, because it’s the place where everyone goes for everything. For example, when the text-to-image generator Stable Diffusion chose to post its models, datasets and demos to Hugging Face, it lit a flurry of user-led activity to modify and improve the model (yielding such masterpieces in their demo space as “a rubber duck making a political speech at a podium” and “Pikachu in Pripyat”).
In short order, AI has rocketed. Large language models (LLMs) have evolved from a backend tech underpinning, say, the suggested short replies Gmail offers you, into something more fantastic (and in some cases, frightening). In rapid succession, text-to-image tools and ChatGPT broadened our ideas about what was possible, and ChatGPT became the fastest-growing app in history.
Lookie-loos and tinkerers are flooding into AI by the millions, crowding a space that has long been a speakeasy frequented only by PhDs and engineers. We’re all using AI now, and this mob of end users is about to exert a lot more influence on the entire space—in ways impossible to predict. In Delangue’s view, anyone invested in the future of AI should be part of the community Hugging Face fosters. “If users don’t understand how this technology is built,” he says, “it creates a lot of risks, a lot of misconceptions.”
In Delangue’s view, the concerns over the rise of a yet-hypothetical artificial general intelligence are misplaced. He aligns with the framework that Andrej Karpathy, the former director of AI at Tesla, put forth in 2017, describing AI as Software 2.0. In this view, AI is a major advance in how the modern world operates, certainly, and one already shaping the economy. But it’s hardly an understudy for God.
“AI is the new paradigm to build all technology. It’s not more; it’s not less. It’s not a new human form. It’s not Skynet or a super sentient being. But it is something massive.”
Clem Delangue
“AI is the new paradigm to build all technology,” Delangue says. “It’s not more; it’s not less. It’s not a new human form. It’s not Skynet or a super sentient being. But it is something massive. It’s bigger than the internet, and it’s bigger than traditional software. It’s going to create new capabilities for technology. In the same way most technology companies write software, most technology companies will write AI.”
This ear-popping growth has intensified another concern for Delangue—that of values. If machine learning lives up to a fraction of its hype and promise, it risks deepening the biases and mistakes of existing technology. To ensure that Hugging Face remains on an equitable path, Delangue has hired Dr. Margaret Mitchell, a former Google ethicist whose research focuses on bias and fairness in algorithms.
As the tools rush into the mainstream, Delangue wants them to remain open and democratic. Every Hugging Face employee is expected to hold up the company’s stated values, which Delangue tries to impart not as edicts, but as Socratic nudges, questions he poses every day in the 300-or-so company Slack channels. Users on the site can also give feedback on results the ML models produce, via the “community” tab. You feel like a model produced results that were biased against you because of your race, religion or gender? “Report it,” Delangue says. He believes the more individuals and companies and regulators understand and can participate in machine learning, the safer the future will be from monopolistic control.
Delangue understands that the sheer speed of AI adoption almost guarantees a degree of chaos, which is, in part, why he isn’t after explosive growth for Hugging Face. “Our main goal is not so much to build a big company or to make money,” he says. “I’m most excited about the potential for change and reinventing new rules, new ways of doing things. And the opportunity to use this moment in AI as a reset button, in a way, to do things differently, to push different values.”
Get the best stories from the Sequoia community.
Last summer, when Delangue was in Paris for his friend (and Hugging Face investor) Ludovic Huraux’s wedding, the two enjoyed dinner at Huraux’s home in the 2nd Arrondissement. Over drinks (wine for Huraux, water for Clem), the founders asked each other: Does money lead to happiness? Huraux admitted that for him, ever more money did not make him happier. Delangue said that he doesn’t think money changes much, because what makes him happy is the work itself. “He’s quite different from other successful CEOs I’ve met,” Huraux says. “What he really loves is to create this community. This philosophy to see his business growing is a way for him to express himself in the world.”
By way of a comparison, Huraux invokes Albert Camus, a favorite philosopher of Delangue’s. And here we find that to understand Delangue, you must take a short detour through hell. There you’ll observe a mythological mortal named Sisyphus. A bit of a trickster, Sisyphus angered the gods by cheating death. They condemned him to roll a boulder up a mountain, on repeat, forever.
In Camus’ read (made famous in his essay Le Mythe de Sisyphe) Sisyphus labors in genuine pain. Yet he nonetheless experiences lightness, even joy, in the moments after the stone rolls back down the mountain. He has no goal but the work; no gods or peers to impress; no money to make; no scorecard of any sort. And as he once again lays hands on that boulder, he finds fulfillment in la lutte elle-même, the struggle itself.
Clem sees a bit of himself in the eternal work of this rule-breaker. “The last sentence in the book,” Delangue tells me, “says you should picture Sisyphus happy. If we compare that to building a company, it means that happiness doesn’t come from being successful. It actually comes from the everyday struggle and endeavor of building one feature after another. Convincing one community member. One step after another. And even the most mundane tasks, you can find joy in them.”
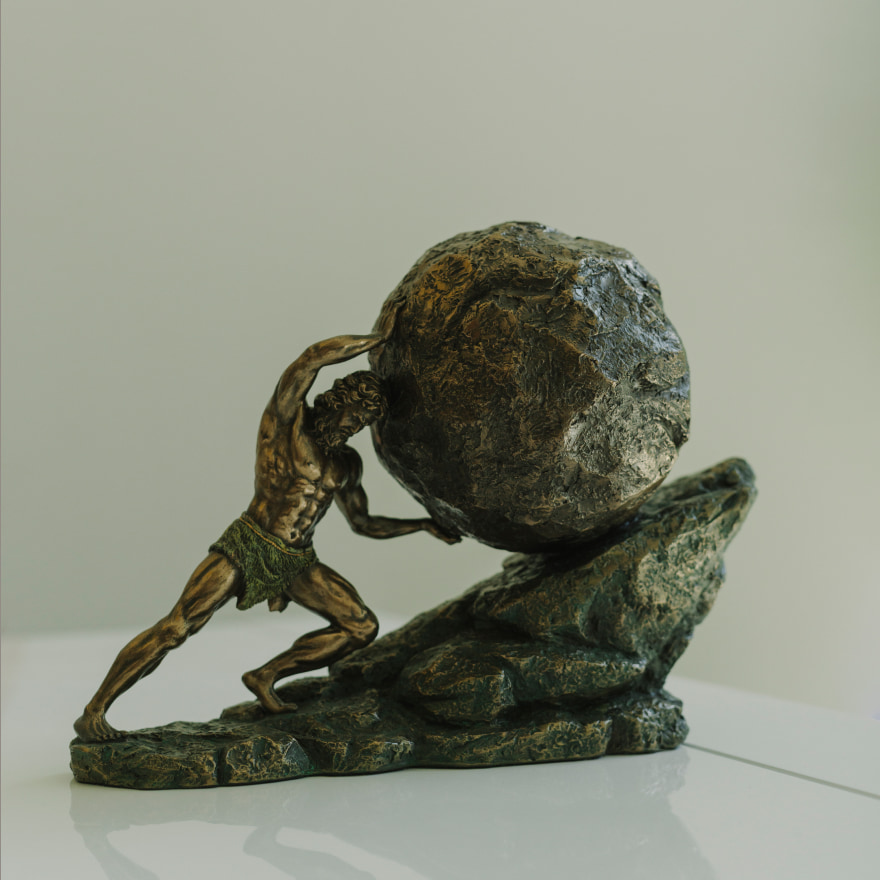
In March of 2023, Delangue tweeted that he was holding an impromptu get-together with Hugging Face users on an upcoming trip to San Francisco. Within a few hours, more than 400 people registered using the password he’d shared in a tweet: ossftw (open-source software for the win). By the following week, the cat was officially out of the bag. “The buzz is real, and we’ve just expanded capacity of the meetup to match it (from 300 to 1,000!),” the main Hugging Face account tweeted. Two weeks later, an engineer at Hugging Face added: “What’s better than a meetup of 2000 people? A meetup of 2500 of course!”
You may be asking how anyone holds a get-together for anywhere between 400 to 2,500 people on short notice, in San Francisco. The answer includes: Signing a venue as late as possible. Haggling with multiple caterers who drop out, looking into the number of pizzerias within a 25-mile radius, then eventually settling for a couple of thousand macarons to feed everyone. Also, you’ll want to have someone sew a Hugging Face mascot costume in just 24 hours. Finally, you’ll need to call every llama farm in California (a nod to LLaMA, or Large Language Model Meta AI) and then check for state and city regulations about camelids in public spaces.
By the time of the event, it had earned the moniker “Woodstock of AI” (and the accompanying #WoodstockAI hashtag). Some 5,000 people (and three llamas) showed up to the Exploratorium science museum. Videos from the event capture something reminiscent of a rave crossed with a robotics competition. AI companies jammed into booths. One of them, an AI-in-education company that formed during a hackathon just a few days earlier, had their public launch at the event. Delangue danced with a mascot in bright yellow tights and an enormous Hugging Face emoji body. He gave a shout-out to everyone who has pushed AI forward (so, pretty much the whole crowd). “This event is a celebration of the power of open source,” Delangue told them. “It’s really important for us to remember in AI that we are where we are because of open science and open source.”
If you didn’t make this one, don’t sweat it. Delangue loves bringing people together, so you can be sure this event won’t be his last. And as always, when the next one comes around, everyone’s invited.