Generative AI Is Exploding. These Are The Most Important Trends To Know
This year’s AI 50 list shows the dominance of this transformative type of artificial intelligence, which could reshape work as we know it.
When we launched the AI 50 almost five years ago, I wrote, “Although artificial general intelligence (AGI)… gets a lot of attention in film, that field is a long way off.” Today, that sci-fi future feels much closer.
The biggest change has been the rise of generative AI, and particularly the use of transformers (a type of neural network) for everything from text and image generation to protein folding and computational chemistry. Generative AI was in the background on last year’s list but in the foreground now.
The History of Generative AI
Generative AI, which refers to AI that creates an output on demand, is not new. The famous ELIZA chatbot in the 1960s enabled users to type in questions for a simulated therapist, but the chatbot’s seemingly novel answers were actually based on a rules-based lookup table. A major leap was Google researcher Ian Goodfellow’s generative adversarial networks (GANs) from 2014 that generated plausible low resolution images by pitting two networks against each other in a zero sum game. Over the coming years the blurry faces became more photorealistic but GANs remained difficult to train and scale.
In 2017, another group at Google released the famous Transformers paper, “Attention Is All You Need,” to improve the performance of text translation. In this case, attention refers to mechanisms that provide context based on the position of words in text, which vary from language to language. The researchers observed that the best performing models all have these attention mechanisms, and proposed to do away with other means of gleaning patterns from text in favor of attention.
The eventual implications for both performance and training efficiency turned out to be huge. Instead of processing a string of text word by word, as previous natural language methods had, transformers can analyze an entire string all at once. This allows transformer models to be trained in parallel, making much larger models viable, such as the generative pretrained transformers, the GPTs, that now power ChatGPT, GitHub Copilot and Microsoft’s newly revived Bing. These models were trained on very large collections of human language, and are known as Large Language Models (LLMs).
Although transformers are effective for computer vision applications, another method called latent (or stable) diffusion now produces some of the most stunning high-resolution images through products from startups Stability and Midjourney. These diffusion models marry the best elements of GANs and transformers. The smaller size and open source availability of some of these models has made them a fount of innovation for people who want to experiment.
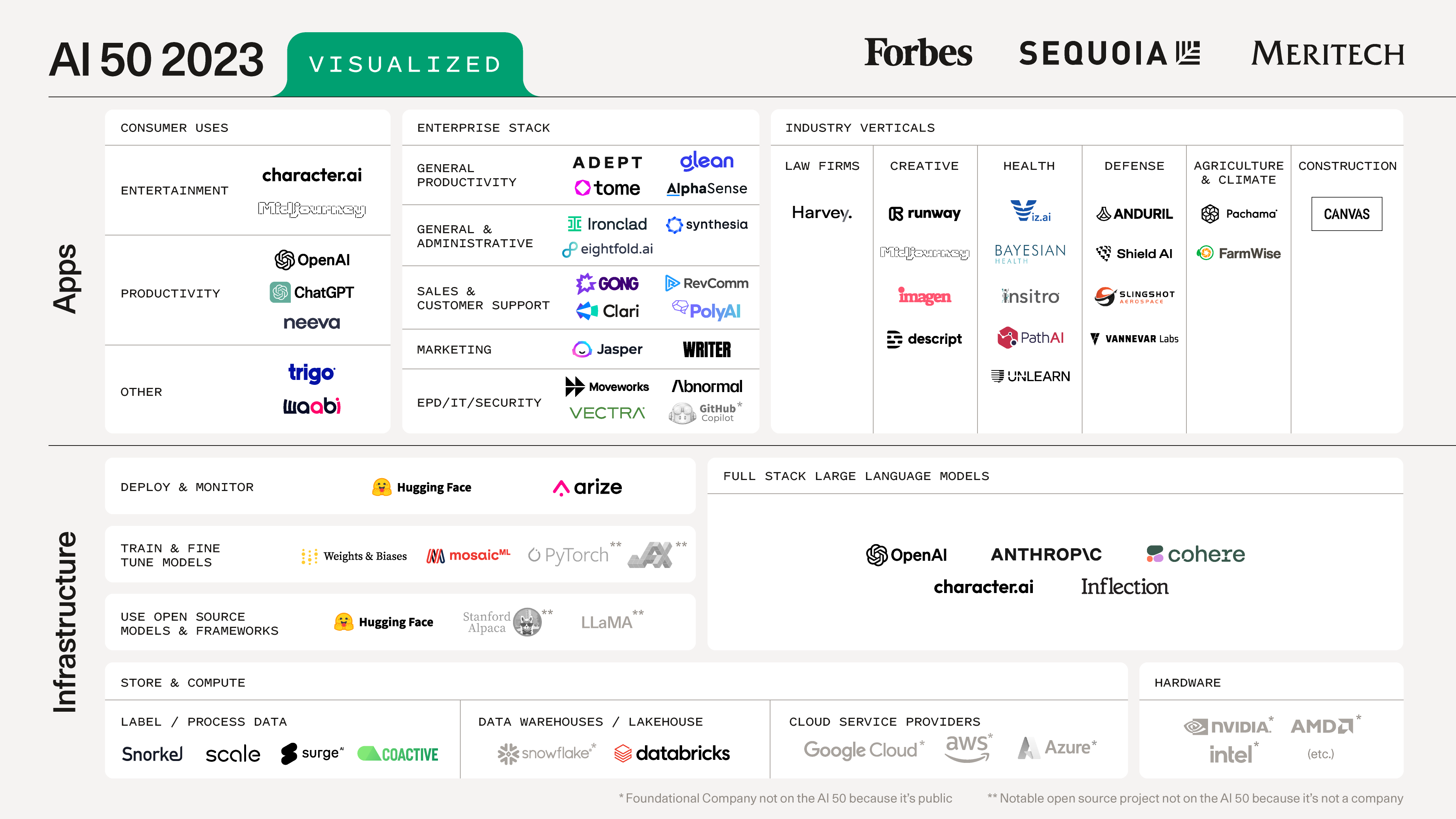
Four trends in this year’s list
Generative AI Infrastructure: OpenAI made a big splash last year with the launch of ChatGPT and again this year with the launch of GPT-4, but their big bet on scale and a technique called Reinforcement Learning with Human Feedback (RLHF) is only one of many directions LLMs are taking. Anthropic and their chatbot Claude use a different approach called Reinforcement Learning Constitutional AI (RL-CAI). The CAI part encodes a set of human-friendly principles designed to limit abuse and hallucination in the outputs. Meanwhile Inflection, a secretive startup founded by DeepMind’s Mustafa Suleyman and Greylock’s Reid Hoffman, is focusing on consumer applications.
And these are just the high-profile entrants on the closed source side. In the world of open source, Hugging Face has become the go-to platform for developers that want to train their own models or fine-tune existing ones. Along with Stability’s open source offerings, Hugging Face also hosts recent state of the art models like Facebook’s LLaMA and Stanford’s Alpaca.
Predictive Infrastructure: During the Gold Rush many individual prospectors went bust, but the people selling the picks and shovels made out just fine. This is why investors often focus on novel infrastructure companies during technology shifts. AI in its many forms is about prediction, so let’s call this new category predictive infrastructure.
The largest of these infrastructure companies host the massive amounts of data needed for enterprise AI applications in a format that facilitates all sorts of data pipelines. Databricks has distinguished itself from Snowflake, a notable incumbent in the space, by being specifically designed for the needs of AI/ML data teams.
Because data labeling, cleaning and other processes are so critical to model training, there are now four companies in this bucket on this year’s list: Coactive, Scale, Snorkel and Surge, up from just one last year (Scale). Two other new entrants to the AI 50, MosaicML and Weights & Biases, specifically help AI practitioners train and fine-tune models. Arize and Hugging Face also make it easy to deploy models at scale.
Generative AI Applications: Midjourney and Stable Diffusion benefited from their virality on social media, planting generative AI in the center of popular culture. Then ChatGPT captured the world’s attention and became the fastest product to attain 100 million users. While Google played catch up with its Bard chatbot, Neeva became the first generative AI-native search engine.
Since LLMs were primarily designed to generate text, generative writing apps are a fast-growing category. Two of these applications are on this year’s list: Jasper, which uses GPT-4 to help marketing copywriters, and Writer, which has trained its own proprietary model and is focused on enterprise use cases. As language models have become more capable, they are able to handle more complex applications, like legal text. Harvey is using GPT-4 to do associate level legal work at law and other professional services firms, while Ironclad has automated many contract processes for in-house legal teams.
Generative AI is inherently creative, so it’s natural to see a lot of innovation in other creative fields. Runway generates, edits and applies effects to video that met the quality bar for the Oscar-winning team behind Everything Everywhere All at Once. Descript focuses on both podcast and video workflows, using generative AI to make the editing process less laborious. ChatGPT, Bing and Bard are general purpose chatbots, but crafting bespoke chatbots is a nascent creative space powered by Character.AI, founded by one of the authors of the original Transformer paper, Noam Shazeer.
Making Powerpoint decks is as close as many people get to being creative at work, but new generative AI apps like Tome make it easy to design beautiful presentations that bring your ideas to life with only text prompts. Another take on work productivity comes from Adept, which has built an action model, ACT-1, that’s trained on how people interact with their computers. Its goal is to eventually automate some of the searching, clicking and scrolling you have to do now to get tasks done.
Predictive AI applications: Another useful way to use AI’s predictive power is to detect anomalies and then find ways to mitigate them. Abnormal Security, for instance, analyzes a company’s cloud email environment to identify phishing attempts and other threats and remove malicious emails. On the medical front, Viz.ai quickly surfaces patient imaging that should be reviewed by a specialist, and coordinates the care team to improve outcomes for patients with stroke and other time-sensitive conditions.
On AI’s horizon
By the time next year’s list rolls out, I believe generative AI and LLMs will still be dominant. But the landscape is changing quickly and there are big opportunities for companies that can move with it. Here are three things to look out for in the coming year:
- The Infrastructure layer is very “fat,” with the biggest companies currently in the space offering models and cloud services. This will change as companies building applications learn how to capture value.
- LLM usage will mature with some companies strongly favoring buying AI models from cloud APIs and others just as passionately wanting to build their own. Many have predicted that startups will graduate from APIs to their own smaller, more efficient models as they grow. Companies with large and unique data stores will see clear advantages to training their own models as moats. Bloomberg’s recent announcement of their bespoke LLM, which is focused on financial language processing, is a great case in point.
- The fast but far-sighted will survive as this wave of AI unleashes massive societal changes. The ability to adapt, pivot and take advantage of unforeseen opportunities will be key. Because this technology has huge potential to transform work, the only constant will be change.
Generative AI has turned many assumptions on their head. In the early days we thought AI would replace manual work, but robotics turned out to be harder than some parts of cognitive knowledge work. And, equally surprising, the approximate nature of generative models makes them better than expected at creative work and less than completely trustworthy on rote, mechanical tasks.
Achieving artificial general intelligence, that futuristic self-learning system that some fear could threaten humanity, is still a moving target. But there can be no doubt that the progress of large language models over the past year has been transformative—and their applications increasingly general. We’re seeing new use cases every day that demonstrate how AI will change the way we work, create and play.
This story was originally published on Forbes.com.