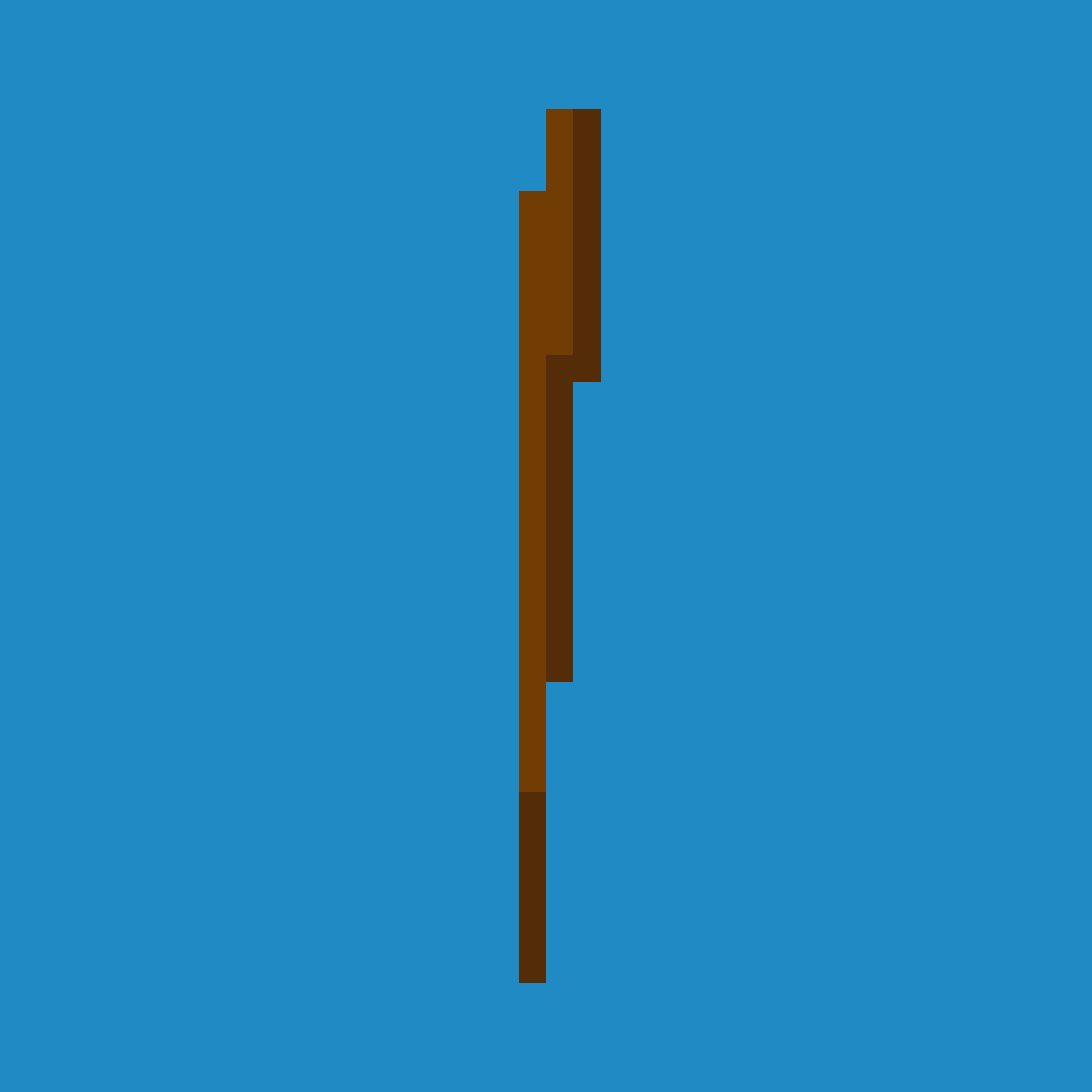
Bringing Generative AI to Healthcare
Healthcare is a massive market rife with administrative inefficiencies. Of the over $4T in annual spend in the U.S. alone, $300B of that is administrative opex, mostly in repetitive, labor intensive processes. Generative AI is especially well suited to attack the labor costs of this services-heavy industry. As we have seen in legaltech, LLMs may unlock growth and disruption in a traditionally difficult vertical for software. Right now, the main applications of this technology target cost saving and automation in back office operations and making workflows more efficient for frontline staff, but the dream is for generative AI to dramatically improve patient outcomes.
We are already seeing innovative companies attack specific use cases, such as medical scribing, patient engagement and other workflows like prior authorization—and new opportunities are being discovered every day. The battle is already brewing. Faced with the familiar incumbents’ advantages in distribution, new entrants must lean on all their speed, ambition and creativity to break through, succeed and endure.
Market Background
Over the past decade, many new healthcare software companies confronted unfavorable market dynamics. Providers operate with razor thin margins and are often unwilling to spend on the promises of long-term cost efficiencies. Payors also suffer low margins and are a concentrated buyer group, with the top 5 players commanding more than 50% market share. These organizations can be slow moving and sales cycles can be incredibly long, creating roadblocks for upstarts.
It is no surprise that the biggest success stories in digital health have largely sidestepped these barriers by:
- Benefiting from one-time regulatory changes (Epic, Cerner, Aledade)
- Focusing on healthcare delivery within specific verticals of care (Maven for fertility, Livongo for diabetes, Hinge/Sword for MSK, Lyra/Headway for mental health)
- Delivering a product experience adjacent to care (Zocdoc to help patients find doctors and book appointments, Doximity to help doctors connect with each other)
On the other hand, success in attacking core healthcare operations are few and far between, with the rare bright spots generally emphasizing revenue enablement over cost reduction (e.g., Viz, Cedar). The picture is even dimmer on the payor side. Frustrated with the intransigence of payors to adopt new technology, some startups have marched into the payor market instead, often with similarly disappointing outcomes.
GenAI tackles these constraints directly. It overcomes the buyers’ “poverty trap” by delivering large and immediate value, while maintaining robustness to unstructured data and operating environments. Its novelty factor and recognizable impact help to galvanize buyers, especially those who hope to appear innovative among peers. Most importantly, new entrants can leverage genAI to get a foot in the door and a chance to attack the broader healthcare software stack. The companies in our landscape represent these opportunities across six broad categories of front and back office operations.
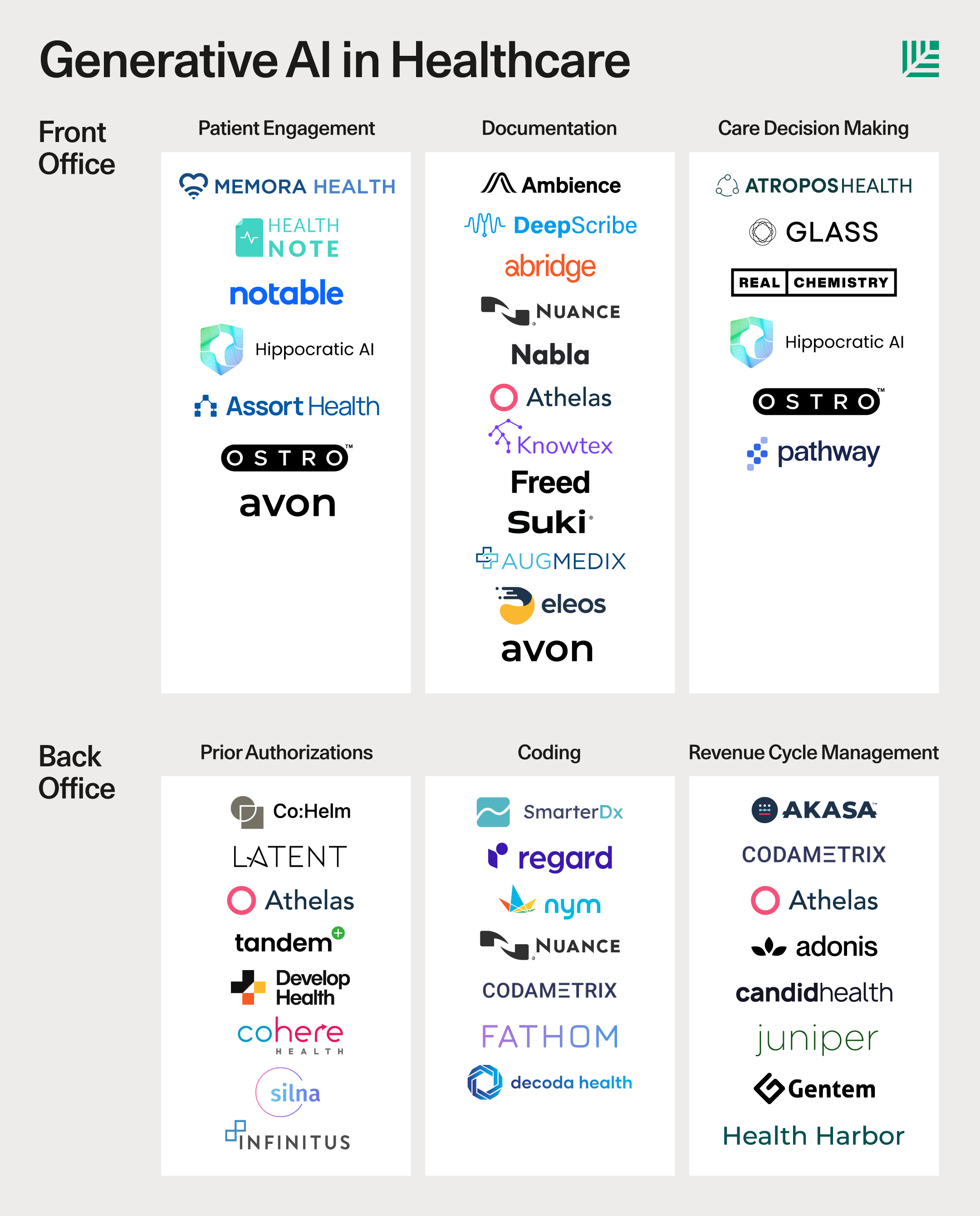
Front Office
Patient-facing workflows are well-suited to LLMs because they are natural language interfaces that require the flexibility to address a wide range of conditions and special cases. There is also the potential for alignment here between care providers, payors and pharma companies, creating vectors for monetization.
Patient Engagement
There are 3 parts to patient engagement—pre-consultation discovery, patient intake and post-consultation care adherence. Discovery and intake are good fits for generative AI, which can access unstructured data to reduce search friction and help patients find the right provider more easily.
The best opportunity here is to improve post-visit care adherence. Patients take only half of the medication prescribed for chronic conditions leading to more than $100B in unnecessary health expenses. The solution can be as simple as automating the texts and calls that remind patients to go to follow up appointments, take medications and answer their basic questions. These tasks are currently done by legions of nurses and case managers. Cleveland Clinic, for example, gets 6M calls every month. Because payors bear the cost of non-adherence from aggravated ailments while pharma loses revenue for drugs not taken, there may be creative go-to-market angles here that startups can leverage.
Documentation
Patient-doctor interactions during consultations generate a load of manual process work, particularly transcribing these conversations into EHR fields and coding them appropriately. This taxes already overworked medical professionals and is often blamed for elevated professional burnout rates. There are an estimated 100K medical scribes today, up from 20K in 2016. With an average spend of $40-50K per scribe per year, this seemingly narrow use case costs at least $4B, exclusive of physicians’ opportunity costs.
In the long term, players here have strong platform potential. They can deepen their features on the captured data, providing better referencing and workflows and eventually becoming a first-class system of record. Some documentation companies are already expanding downstream into areas such as coding and billing.
This is not a new insight, but there is a clear “why now.” The last generation of startups fell short because the tech was not ready, but the problem lends itself well to today’s LLMs, particularly Whisper and GPT4 models. Ironically, the risk now is that it is too easy and the tech will almost surely commoditize. In the market of smaller health systems and clinics, startups will need to go beyond the scribing wedge to create an all-in-one suite for provider operations.
Clinical Decision Making
As shown in legaltech, genAI can provide an interface to organize, retrieve and synthesize complex medical facts, notes and research. Physicians have traditionally been reluctant to embrace new workflows, but other use cases are potentially open to attack. For instance, one could envision LLMs empowering physicians to query a vast corpus of drug information or providing more personalized care for a patient.
Back Office
Much of the back office workload stems from the conflicting incentives between payors and providers. Providers often get paid for the service, rather than the outcome. Payors are naturally skeptical of what providers represent as necessary and would rather not pay for a service or drug. As a result, payors force providers through complex frameworks and arduous processes to justify their reimbursement requests and deny more than 1 in 10 claims. Payors are also burdened by the need to manually review and adjudicate claims. Both sides thus employ thousands of nurses and administrative staff to handle these tasks.
Prior authorization
Prior authorization is the arduous process insurance companies impose on physicians to seek approval before they can prescribe certain drugs to a patient or schedule certain procedures. In 2021, physicians submitted more than 35 million prior authorization requests to Medicare Advantage payors, of which 2 million were denied. AI-enabled automations arm the providers, patients and pharma companies—whose incentives are all aligned—against this death by administration. LLMs have been able to generate prior authorization forms with remarkable accuracy out of the box, which is why so many startups have started here.
There is a risk that this need may get regulated away. As the number of prior auths have grown so egregiously over the last few years, regulatory intervention looks increasingly likely. New policies that reduce the burden of prior auths overall would dramatically reduce the value of these products. And, as with AI-scribes, the technology to generate a prior authorization form is also fairly commoditized, so companies have to build out additional workflows to endure.
Coding
Medical coders read physician notes and look at labs to identify the right code for the diagnosis and procedure. These codes are then used for insurance billing. The medical coding market in the U.S. is worth around $21B, comprising about 35K medical coders. Despite all that labor, almost $20B of revenue is lost by U.S. hospitals annually due to coding errors, which has led to a cottage industry of local consulting firms that help providers “discover” missing revenue.
While most of the traditional solutions are rule-based, multimodal LLM models can collate unstructured physician notes, lab panels, and imaging to determine the right diagnosis codes. Automation can also reduce the administrative back and forth with payors and will be a natural lead into the massive opportunity that is revenue cycle management. Incumbents in the documentation space will also have a formidable advantage here.
Revenue Cycle Management
Medical billers create and submit a medical claim to the payor once they get the codes for the procedures/office visit. While claim submission is automated, following up on them is not. The combination of investigating and appealing rejected claims, verifying eligibility and benefits of all treatments and dealing with payors is probably the most significant administrative headache for provider systems. Eleven percent of all healthcare insurance claims were denied in 2022.
There are two ways to attack the RCM market: workflow automations or handling the billing itself. Automation comes with some tech risk and significant amounts of incumbent advantage (e.g., UiPath incorporating LLMs), while billing suffers from protracted sales cycles. The best entrypoint may be to expand into RCM over time from adjacent use cases.
Conclusion
Disruption in healthcare has historically been difficult and the windows of opportunities fleeting and narrow, but generative AI may finally provide the unlock. We are excited to meet teams who dare to reimagine the next decades of healthcare.
If you are a founder working with generative AI to improve healthcare workflows, I’d love to hear from you. Please reach out to josephine@sequoiacap.com.